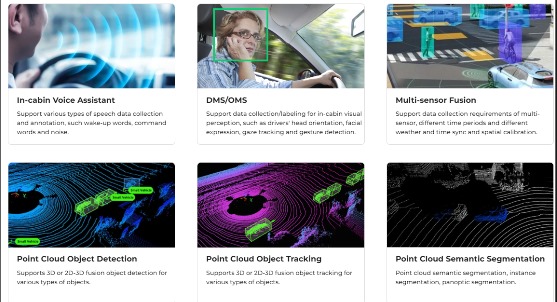
Artificial Intelligence (AI) is transforming industries, from healthcare and finance to manufacturing and entertainment. AI technologies are driven by the ability to process vast amounts of data and extract meaningful patterns from it. However, the foundation of any successful AI system is its data. A high-quality dataset for AI is crucial to improving the performance and accuracy of algorithms. This article explores the importance of quality datasets in advancing AI technologies, examining how they impact the development and deployment of AI models, and why investing in the right datasets is essential for AI’s continued evolution.
Understanding the Role of Data in AI Development
AI algorithms rely heavily on data to learn and make predictions. Machine learning (ML), a subset of AI, involves training algorithms on large datasets to identify patterns, make decisions, and improve over time. In simpler terms, data acts as the fuel for AI systems. Without it, AI models would have no basis on which to make decisions or predictions.
The quality of a dataset is a determining factor in how well an AI model performs. A dataset for AI is often composed of labeled or unlabeled examples that represent real-world scenarios. These examples could include anything from images and text to sensor data and financial transactions. The more relevant, accurate, and diverse the data, the better the AI model can learn and generalize.
Key Characteristics of a Quality Dataset for AI
When considering a dataset for AI, there are several factors that contribute to its quality. These characteristics are essential to ensuring that the AI system can function optimally and produce accurate results.
1. Relevance
For an AI model to make accurate predictions, the dataset must closely align with the problem the AI is trying to solve. For instance, if the goal is to develop an AI system for medical diagnostics, the dataset should primarily consist of medical images, patient records, or health-related data. Irrelevant data could introduce noise and make it harder for the model to learn the correct patterns.
2. Accuracy
The accuracy of a dataset is one of the most critical factors in AI development. If the data contains errors or inconsistencies, these flaws will be carried over into the AI model. For example, if a dataset contains mislabeled data points, the model may learn incorrect associations, leading to poor performance. Ensuring data accuracy involves thorough cleaning, verification, and validation processes to eliminate errors.
3. Diversity and Representativeness
To avoid bias and ensure that an AI system can generalize across various scenarios, a dataset must be diverse and representative of the real-world situation. If a dataset only contains data from a specific demographic, region, or condition, the AI model may not perform well when exposed to new, unseen data. For example, facial recognition systems trained on a dataset with limited racial or ethnic representation may exhibit biased results. Ensuring diversity in datasets is crucial to mitigating such risks and improving the fairness of AI systems.
4. Size and Scale
The volume of data in a dataset is another important consideration. Larger datasets tend to improve the performance of AI models by providing more examples from which the algorithm can learn. However, it’s not just about having more data; it’s also about having a sufficient amount of high-quality data. Too little data may lead to underfitting, where the model cannot generalize well to new data. On the other hand, excessively large datasets with irrelevant or noisy data can slow down the training process and lead to inefficiencies.
5. Consistency
Data consistency is essential for training AI models that perform reliably over time. If the dataset is inconsistent, with varying formats, units of measurement, or structures, it can confuse the AI algorithm and hinder its learning process. For example, in a dataset for natural language processing (NLP), inconsistencies in spelling, punctuation, or grammar could affect how well the AI system understands and processes text.
The Impact of Quality Datasets on AI Technologies
Quality datasets are fundamental to the success of AI technologies. Below are some key ways in which a well-curated dataset for AI can drive advancements in various AI fields.
1. Enhancing Model Accuracy and Reliability
AI models rely on accurate data to produce meaningful results. When trained on high-quality datasets, AI systems are better able to detect patterns, make accurate predictions, and handle complex tasks. For example, in autonomous vehicles, a high-quality dataset of road conditions, traffic patterns, and pedestrian behavior can significantly improve the vehicle’s ability to navigate safely and efficiently.
2. Fostering Innovation and New Applications
A diverse and expansive dataset for AI opens the door to new innovations and applications. As AI models are exposed to a wide variety of data, they can uncover previously unseen relationships, leading to groundbreaking discoveries. For instance, in the field of drug discovery, AI models trained on diverse datasets of chemical compounds, biological information, and genetic data have the potential to identify novel drug candidates and treatment methods.
3. Reducing Bias and Ensuring Fairness
One of the most significant challenges in AI is ensuring fairness and eliminating bias from algorithms. A dataset for AI that is biased or skewed can lead to AI systems that perpetuate discrimination or unfair outcomes. High-quality datasets, when properly curated, can help mitigate these biases. For example, by ensuring that a facial recognition system is trained on a diverse dataset, developers can reduce racial bias in recognition and improve the system’s fairness.
4. Improving Model Generalization
AI models must generalize well to unseen data in order to be effective in real-world applications. A high-quality dataset ensures that the AI model is exposed to a wide range of scenarios, helping it generalize better. For example, in the financial industry, an AI trained on diverse datasets of market conditions, customer behavior, and economic indicators can adapt to changing market trends and make accurate financial predictions.
5. Supporting Real-time and Continuous Learning
In dynamic environments where data is constantly changing, AI systems need to be able to adapt to new information. High-quality datasets enable continuous learning by feeding AI models with up-to-date and relevant data. This is particularly important in areas like cybersecurity, where AI models must learn from new threats and adapt to evolving attack patterns in real-time.
Challenges in Obtaining and Maintaining Quality Datasets
While the importance of quality datasets is clear, obtaining and maintaining them can be challenging. The process of curating a high-quality dataset for AI involves data collection, cleaning, labeling, and validation, all of which can be resource-intensive. Furthermore, maintaining data quality over time is an ongoing challenge, especially as data evolves and new data sources emerge.
Some of the key challenges in working with datasets for AI include:
1. Data Privacy and Security
Data privacy is a critical concern, especially when working with sensitive data such as healthcare records or personal information. Ensuring compliance with data privacy regulations like GDPR and HIPAA is essential to protect individuals’ privacy while still enabling the development of effective AI models.
2. Data Labeling and Annotation
Labeling and annotating data is often a time-consuming and costly process. For supervised learning, labeled data is required for training, but labeling large datasets accurately can be a significant challenge. Mislabeling can lead to biased or inaccurate models.
3. Data Imbalance
Many real-world datasets are imbalanced, with some classes underrepresented. This can lead to poor model performance, particularly in classification tasks. Addressing data imbalance through techniques like oversampling or synthetic data generation is crucial to achieving high-quality results.
Conclusion
In conclusion, quality datasets are the cornerstone of AI development. They directly influence the accuracy, reliability, fairness, and generalization capabilities of AI models. As AI technologies continue to advance, investing in diverse, accurate, and representative datasets will be essential for unlocking new possibilities and improving the effectiveness of AI systems across various industries. A well-curated dataset for AI is not just an asset—it’s a necessity for pushing the boundaries of what artificial intelligence can achieve.